By Nana Yaa Asante-Darko, Sustainability Finance Research Analyst, Entelligent
When it comes to data analytics, the business world today finds itself in a paradoxical position. Big data and machine learning have given commercial organizations more power to read markets — forward and backward — than they have ever had. In many fields, they have more power than they might ever have imagined they would have. However, data and machine learning power are considerably less valuable if the output cannot be “read” and understood by human decision makers. As climate risk measurement and management become mainstream in business, the accessibility of insights derived from climate data is more paramount than ever.
Current Limitations
Climate Risk Data
The data we use to evaluate climate risk is vast—a diverse mix of estimates (like carbon emissions from 200 years ago) and samples of true populations (like temperature measurements taken around the world). As the diagram below suggests, these examples don’t begin to capture the range and variety of data that goes into modern climate and climate transition analysis. Raw climate data can easily overwhelm its would-be user, even more so in this age of geopolitical turmoil and energy contention. The good news, of course, is that artificial intelligence (AI) and machine learning can process and model this data to provide meaningful information for decision-making.
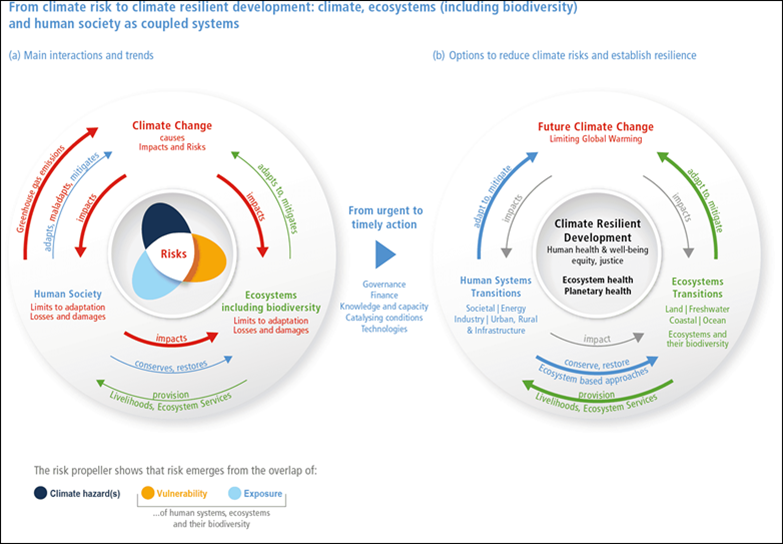
Source: IPCC
Model Interpretability
This brings us to the concept of interpretability. A model can be said to offer interpretability when it enables people—whether business generalists or data scientists—to understand its output. Interpretability is not about eliminating technicality, nor is it about simplification. It’s being able to see the rules of a model’s output in action and having the ability to predict, with some consistency, its future outputs.
Simpler models such as linear regressions with few factors are inherently more understandable, but they can’t make the complex data connections machine-learning models can. As the world is not so straightforward, it’s not possible for simple models to solve its problems. What is possible is to ensure the user community (i.e., investors and capital markets participants) understands how the models function and what they are trying to tell us. Such interpretability is critical.
Going a Step Further
Using version 2.0 of Entelligent’s T-Risk climate transition risk score and model, we can increase our interpretability and see the distribution (and outliers) of Carbon Adjusted T-Risk for each GICS sector.
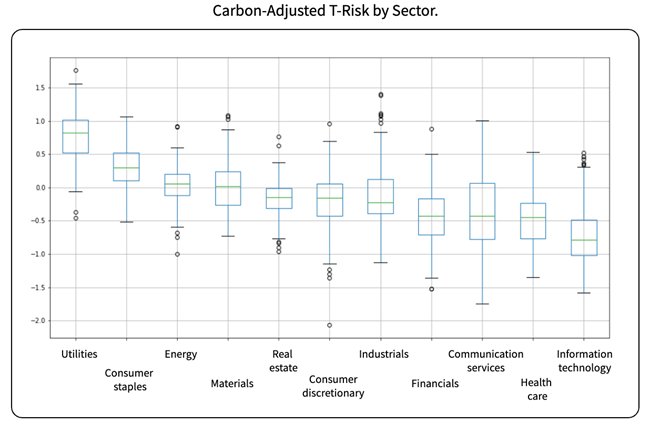
Source: Entelligent
The orderly distribution of the data—like ducks on the surface of a pond—belies the processing that goes on underneath. This is a transparent, patented methodology that begins with global circulation models and integrated assessment models, develops energy market predictions, and then deploys them against 38,000 individual global investment securities through extensive back- and forward-testing—all before applying carbon, as a penalty or bonus. The model makes it easy to compare riskier sectors (i.e., Utilities and Consumer Staples) and positive T-Risk scores on the fly to others that have better risk outlooks. It also gives the user predictive confidence.
The data suggests that climate-sensitive investors today should be more concerned about their exposure to high energy-consuming sectors such as utilities and consumer staples than to the energy sector itself. As shown in the data, the reality is that enterprises need to consume significant energy (much of it from fossil fuels) en route to delivering, for example, the electric vehicles and wind turbines that will ultimately drive consumption down. This is why we see fossil fuel prices continue to rise under a Paris-aligned scenario before eventually falling.
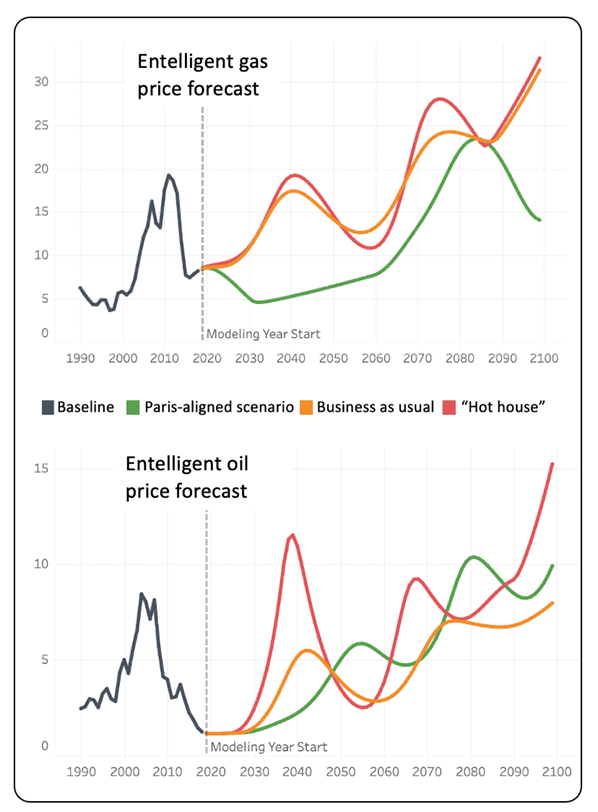
Source: Entelligent
Conclusion
As AI, machine learning, and deeper quantitative methods are increasingly used to generate ESG data and drive sustainability-related investment decisions, asset owners, and asset managers—as well as policymakers and regulators—will need to increasingly gravitate to clearer, more interpretable resources.